Resource cost-aware scheduling problems under uncertainty
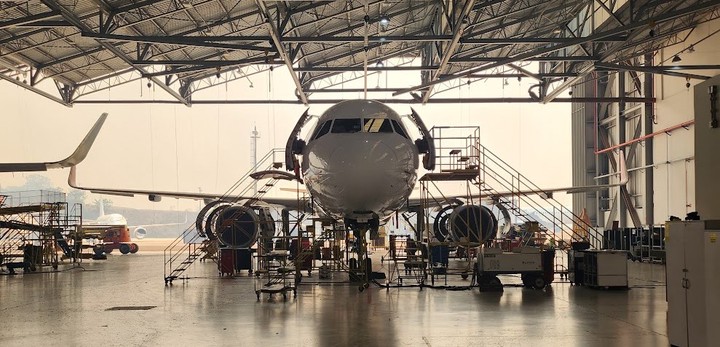
Our project addresses the critical balance between resource consumption and scheduling efficiency in settings where resources are limited or costly, such as astronomical observatories, manufacturing, and maintenance processes. Managing these constraints effectively can lead to substantial performance improvements but requires advanced techniques to weigh the trade-off between consuming resources and meeting scheduling demands. In many cases, resources, such as energy or workforce, are not only limited but also costly, adding an extra layer of complexity to scheduling tasks optimally.
A unique aspect of this research is its focus on scheduling under uncertainty, where key parameters, like job sizes or deadlines, may not be known beforehand. This complexity is particularly relevant to observatories in Chile, where variable factors such as sky quality or observation lengths affect task durations. Through collaboration with some of the largest observatories, we gain access to unique datasets and domain expertise, enabling us to test our methods in real-world scenarios. This approach also opens pathways to apply our findings in other industries that face similar scheduling and resource challenges.
We are developing a comprehensive set of prescriptive analytics tools, including new algorithms with strong theoretical guarantees and benchmarks that address these resource-cost-aware scheduling problems. Our work will involve constructing approximation algorithms using mathematical programming and resource augmentation techniques. We aim to build a publicly accessible repository of benchmark instances—using real-world data and factoring in uncertainties and various scheduling metrics—that will advance research in this field and provide robust, adaptable solutions for diverse applications.
This project is funded by FONDECYT 1231245 grant from ANID.
Project Team:
- PI: Rodrigo A. Carrasco
- Co-PI: Gonzalo Ruz
- Co-PI: Luis Aburto