ClimateDL: Forecasting of extreme temperature events
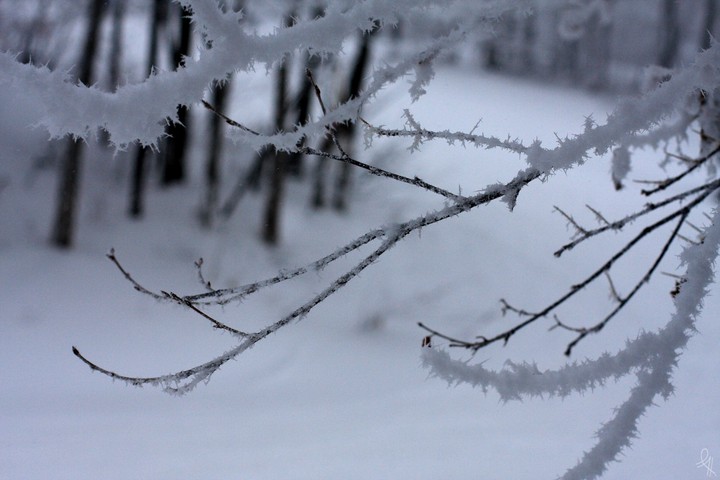
Forecasting extreme seasonal temperature events is especially relevant to society and its ecosystem due to their potentially dangerous impacts. Despite the above, the study of this problem is still very nascent in South America, where most of the methods proposed in the literature correspond to process-based dynamic models and classical statistical methods. Increasing the accuracy of predicting the probability of occurrence of extreme temperatures at the seasonal scale would have huge impacts on our economic life (food production, energy consumption), health, etc.
Innovative approaches are emerging in climate forecasting, using network science and machine learning methodologies. The complex interrelations between temporal and spatial variables in climate time series justify the use of climate networks as an underlying automatic model explanation of the complex physical processes involved. The use of machine learning methods, particularly deep learning architectures, is justified by their success in several forecasting problems.
In this project, we will evaluate the precision of the most prominent deep-learning architectures for our forecasting problem, particularly those implementing spatiotemporal models based on graphs (climate networks). The most promising antecedents for this methodology are several works based on climate networks and the effect of “El Niño” on similar forecasting problems. We expect to propose improvements to these deep learning architectures to increase their precision in our particular problems. For example, changing the scale of nodes, changing the similarity of edges, proposing new architectures that include multiple networks, training the model with augmented data, etc. We will implement and compare the results with classical statistical methods, such as principal component regression technique and Wavelet transform-based methods, among others. Assuming we achieve good predictive quality, we will evaluate the impact of these predictions on some human activities. We will focus on risk analysis of specific infrastructures (telecommunication networks, energy distribution) and on concrete case studies in situations where the considered extreme events have a strong negative impact and for which historical data are available.
This project is funded by grant 22-CLIMAT-02 (AMSUD210012) from ANID.
Project Team:
- International Project Coordinator: Pablo Rodríguez Bocca, Universidad de la República, Uruguay
- Country Coordinator (Chile): Rodrigo A. Carrasco
- Country Coordinator (Argentina): Matilde Rusticucci, Universidad de Buenos Aires
- Country Coordinator (France): Yassine Hadjadj-Aoul, INRIA
- Researchers: (Argentina) Mariana Barrucand, Soledad Collazo, (Chile) Javiera Barrera, Eduardo Moreno, (France) Gerardo Rubino, Sebastián Basterrech, (Uruguay) Madeleine Renom, Diego Kiedanski.